Unlocking Next-Level Efficiency: How RAG Supercharges AI with Your Company's Expertise
Enrich AI with your company’s data for better employee and customer experience using Retrieval-Augmented Generation (RAG).
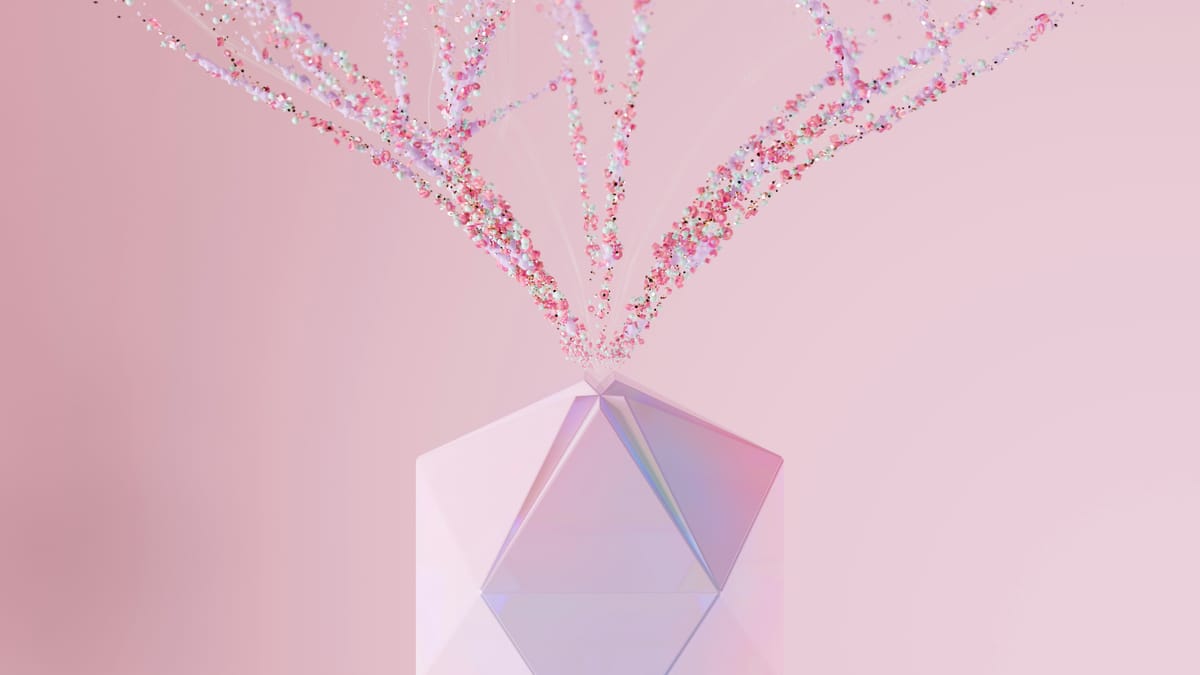
In the modern workplace, employees rely heavily on Large Language Models (LLMs) such as ChatGPT to streamline tasks, access information, and boost efficiency. These LLMs are typically trained on public data up to a certain cutoff date and lack access to a company's internal knowledge.
To provide more contextual and up-to-date responses, one option is to continuously fine-tune models with additional training. However, this is a costly and often impractical solution for most companies. Instead, many organisations are turning to a technique known as Retrieval-Augmented Generation (RAG).
What is RAG?
RAG enhances LLMs by incorporating relevant information into prompts before sending them to the model. This allows the LLM to consider this contextual information when generating responses. At the core of RAG is a vector-based database connected to the company’s internal data sources. Data from these sources are continuously indexed in the vector database as embeddings, which are numerical representations of words, phrases, or entire documents.
How RAG works
- Prompt Submission: An employee submits a prompt.
- Internal Search: The internal search engine analyses the prompt, searches for relevant information in the vector database, and fetches corresponding documents.
- Contextual Addition: The relevant information is extracted from these documents and added to the prompt.
- LLM Processing: The enhanced prompt is sent to the LLM, which generates a more accurate and contextually relevant response.
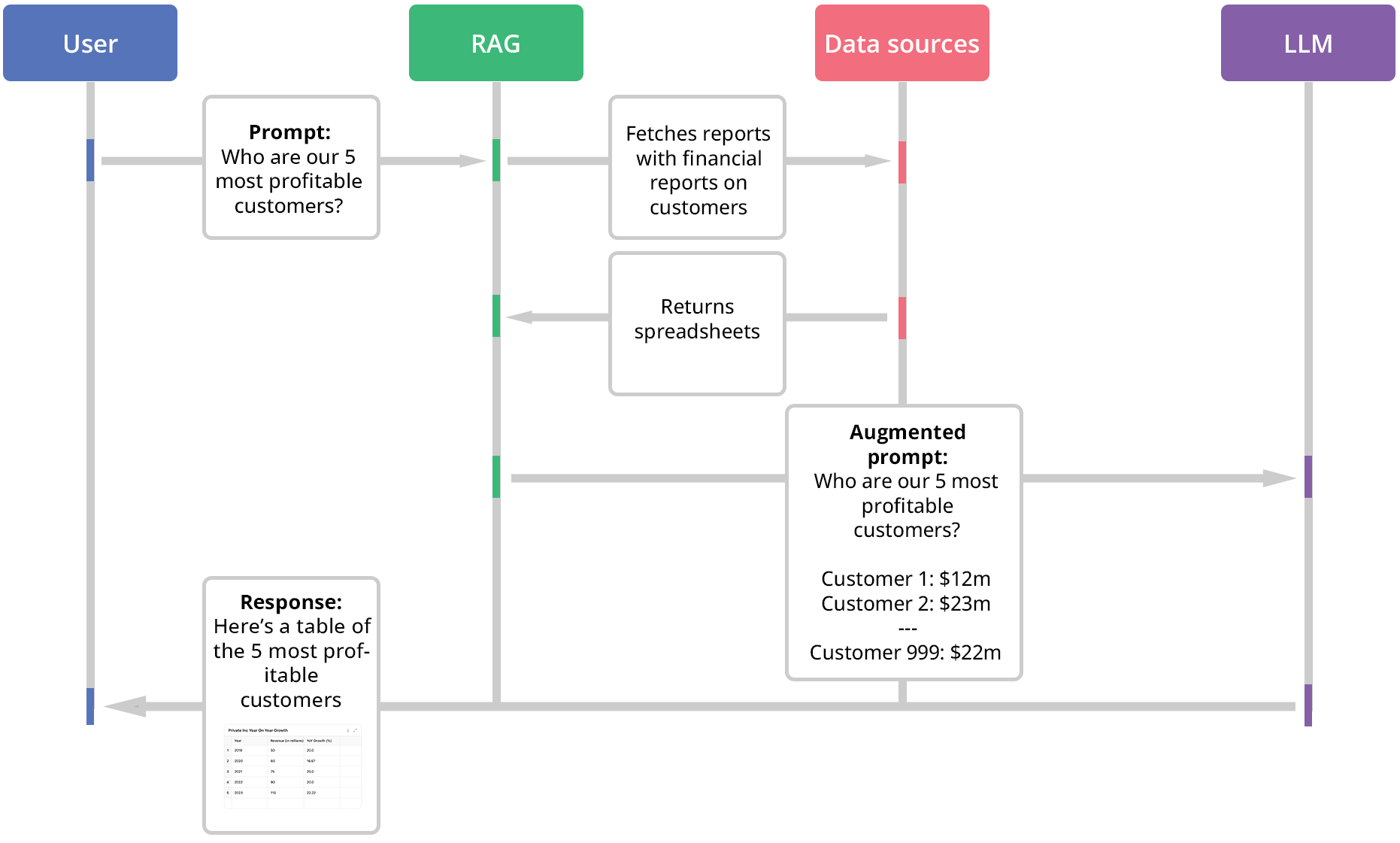
Implementing RAG
There are several ways to implement RAG, depending on a company's needs and constraints:
- Vendor-Supported RAG: Some LLM vendors, like OpenAI, offer built-in RAG mechanisms. Companies can upload files to the vendor's platform, where they are stored and indexed in the vendor's vector database. This is suitable for use cases with limited data and where company policies permit uploading proprietary information to an external cloud.
- Cloud Infrastructure RAG: For companies requiring data to stay within their geographical jurisdiction or cloud infrastructure, cloud providers like Microsoft Azure offer RAG mechanisms that integrate with models like ChatGPT hosted in the customer’s cloud.
- On-Premise RAG: For complex needs or where data must remain on-premise, companies can purchase an on premise RAG tech stack from vendors like Glean or Danswer, or implement it from scratch. Key components include file storage, a vector database like Qdrant for retrieving relevant documents, and a model to extract pertinent information from these documents. Extracted information is then added to the prompt. On-premise models like Meta’s Llama3 can also be utilised.
Ensuring successful implementation
While the concept of RAG is straightforward, implementing it successfully involves considerable complexity. Partnering with an experienced implementation partner can greatly enhance the likelihood of a successful RAG project. Such partners can navigate the intricacies of setting up the tech stack and ensure that the RAG system is optimally configured for your organisation's needs.
By leveraging RAG, companies can significantly enhance the contextual relevance of their LLMs, leading to improved productivity, better decision-making, and a more efficient workforce.