Deploying AI: Safeguard Your Intellectual Property and Stay GDPR Compliant
Discover how to deploy AI technology while safeguarding intellectual property, ensuring data privacy, and staying GDPR compliant.
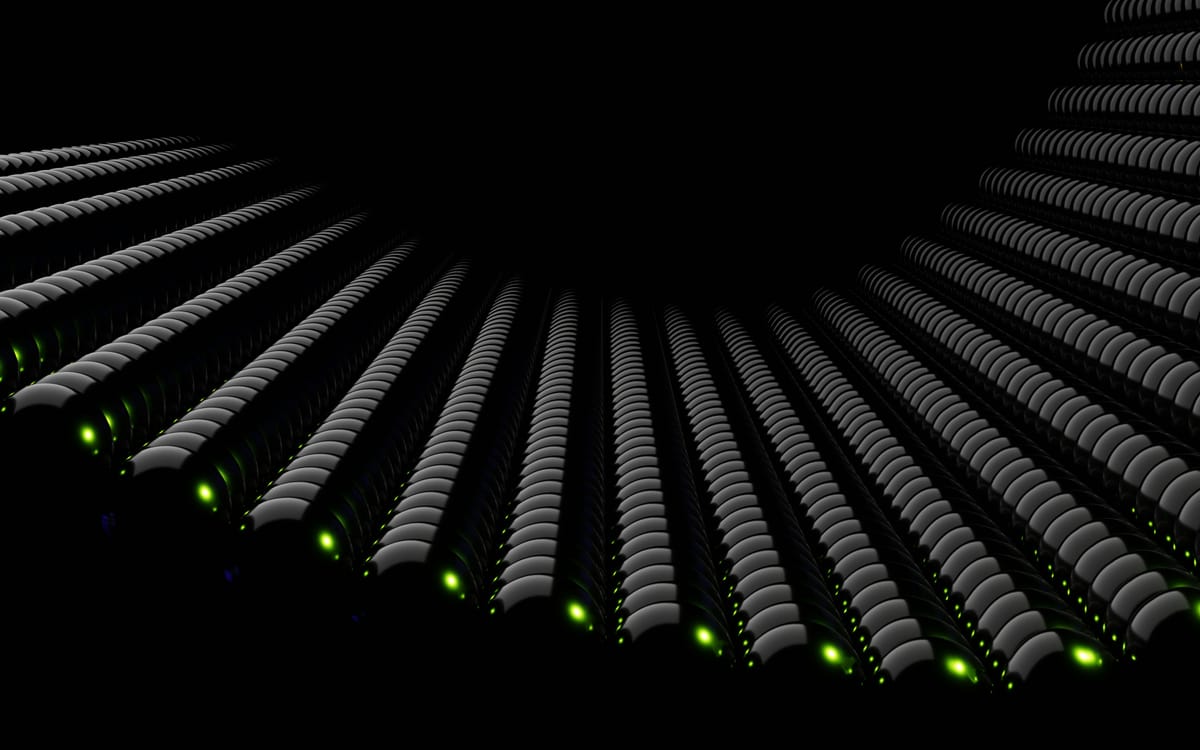
As AI technology becomes increasingly integral to business operations, Large Language Models (LLMs) like ChatGPT and other AI technologies are at the forefront of driving innovation. Sophisticated models power various applications, from customer service chatbots to automated content creation, offering significant value to enterprises. However, deciding how to deploy AI technology is crucial, especially when it comes to safeguarding intellectual property, data privacy, and regulatory compliance.
In this article, we’ll explore three primary deployment options: using a vendor through an enterprise plan, hosting models in your cloud, and hosting them on-premise. We’ll weigh the pros and cons of each approach, focusing on safeguarding intellectual property, data privacy and GDPR considerations.
1. Vendor-managed software as a service
One of the most straightforward ways to deploy AI models is by leveraging a software as a service (SaaS) solution from a vendor through an enterprise plan. In this setup, a model like an LLM is fully hosted and managed by the vendor, who provides access to the model through a web interface, API, or other integration methods.
Some vendors offer the option to fine-tune their models using a company’s proprietary data or, alternatively, enhance the model with company-specific information through Retrieval-Augmented Generation (RAG). While many vendors assure in their terms and conditions that any submitted data will remain private, control over the exact flow of this data including intellectual property and personally identifiable information (PII) is limited. For companies bound by GDPR regulations, this lack of control can be problematic, particularly when it involves sending PII data to the US, which may not be a viable option.
Pros:
- Ease of use: The vendor manages all aspects of the model, from infrastructure to updates, allowing businesses to focus on applying AI without needing in-house expertise.
- Scalability: Vendors typically offer highly scalable solutions, enabling businesses to adjust usage based on demand without worrying about underlying infrastructure.
- Cost-efficiency: Enterprise plans are often subscription-based, allowing businesses to avoid the upfront costs of infrastructure while still accessing advanced AI capabilities.
Cons:
- Data privacy concerns: Using a third-party vendor means your data is processed on their servers, which can raise significant data privacy issues, particularly if sensitive or personal data is involved.
- Limited customisation: Vendor-managed solutions are generally standardised, offering limited customisation to meet specific business or security needs.
- Regulatory compliance: Depending on the vendor’s data handling practices, ensuring GDPR compliance can be challenging, particularly if the vendor stores or processes data outside the EU.
GDPR Considerations: Under GDPR, businesses must ensure that any data processing meets strict criteria, such as obtaining explicit consent and ensuring data is not transferred outside the EU without adequate protections. Using a vendor like OpenAI requires careful scrutiny of their data handling policies and contracts to ensure compliance.
Common SaaS vendors include:
- OpenAI: OpenAI provides access to its advanced generative AI models, including GPT-4, through its API and web interface. Businesses can integrate these models into their applications via an enterprise plan, with OpenAI managing the infrastructure and model updates. OpenAI also offers out of the box (without writing custom code and deployments in your own cloud) options to make their models more contextual by either uploading data to fine-tune them or using Retrieval-Augmented Generation (RAG) mechanisms to add context to prompts on the fly. In both cases, the Terms and Conditions for enterprise customers guarantee that such data will remain private.
- Google Gemini: Google's advanced AI model suite, designed to compete with GPT-4. Gemini can be used as an assistant, similar to ChatGPT, and is also integrated directly into Google products like Gmail, Google Docs, Google Sheets, and other applications.
- Anthropic: A newer player in the field and founded by former OpenAI employees, Anthropic offers AI models such as Claude that focus on safety and alignment. They provide API access to their models, catering to businesses looking for cutting-edge, responsible AI solutions.
- Cohere: Cohere offers natural language processing models and APIs that businesses can integrate into their applications, providing a feature set comparable to the OpenAI platform. They offer various deployment options, including cloud-based SaaS solutions, as well as the ability to fine-tune their models or augment prompts with proprietary data.
- Hugging Face: Hugging Face offers a platform with various models, including LLMs, that can be accessed via APIs. They provide both free and enterprise plans, with the latter offering more robust support and scalability.
2. Hosting in your cloud
Hosting models in your cloud involves deploying them on cloud infrastructure that you control, giving you greater oversight over data, data residency, and security compared to relying entirely on a vendor. Major cloud infrastructure providers offer tools to automate and scale the AI development and deployment process, commonly known as MLOps. These tools enable the easy deployment and fine-tuning of open-source models, and in some cases, closed-source models where partnerships are in place.
Pros:
- Greater control: Hosting models in your cloud gives you control over data storage, processing, and security, reducing the risks associated with third-party access.
- Flexibility: You can tailor the deployment to meet specific business needs, including compliance with regulatory requirements like GDPR.
- Cost management: While hosting in your cloud can be more expensive than using a vendor, it allows for better management of long-term costs through optimised resource allocation.
Cons:
- Technical complexity: Hosting models in your cloud requires significant technical expertise to manage the infrastructure, deploy the model, and ensure it runs efficiently.
- Data privacy responsibility: Although you have more control, the responsibility for ensuring data privacy and security lies entirely with your organisation, which can be challenging to manage.
- Potential for higher costs: While you avoid vendor fees, the cost of maintaining cloud infrastructure and ensuring high availability can add up, particularly as your needs grow.
GDPR Considerations: Hosting in your cloud can be designed to comply with GDPR, especially if you ensure that data is stored within the EU or implement appropriate safeguards for international data transfers. However, you must actively manage these aspects, as you are responsible for compliance.
Common cloud infrastructure providers include:
- Microsoft Azure: Azure offers a comprehensive cloud platform with advanced AI and machine learning tools, including Azure Machine Learning. With Microsoft's stake in OpenAI, Azure provides exclusive access to deploy models like ChatGPT directly within your cloud infrastructure.
- Amazon Web Services (AWS): AWS, through Amazon SageMaker, provides a highly scalable and flexible environment for building, training, and deploying AI models. It offers a wide range of tools and specialized infrastructure, making it ideal for businesses of all sizes.
- Google Cloud Platform (GCP): Google Cloud’s Vertex AI platform offers powerful tools for machine learning and AI model deployment, integrated with Google’s extensive AI expertise. GCP is a strong choice for businesses seeking cutting-edge AI capabilities and seamless integration with other Google services. Vertex AI also offers access to Gemini models from Google.
- IBM Cloud: IBM Cloud, with its Watson platform, provides enterprise-grade AI tools for natural language processing and machine learning. It’s particularly well-suited for industries requiring high levels of data security and compliance, such as healthcare and finance.
- Oracle Cloud Infrastructure (OCI): Oracle Cloud Infrastructure is optimized for high-performance computing and AI workloads, with a strong focus on enterprise applications. OCI is ideal for businesses looking to integrate AI with their existing enterprise systems, such as ERP and CRM solutions.
3. Hosting on-premise
On-premise hosting involves deploying models entirely within your organisation’s own data centres. This method offers the highest level of control and security but requires substantial investment and technical expertise.
Pros:
- Maximum data control: On-premise hosting ensures that all data stays within your organisation, offering the highest level of data security and privacy.
- Customisation: You can fully customise the deployment to align with your specific security protocols, business needs, and compliance requirements.
- No third-party dependency: By hosting on-premise, you eliminate reliance on external vendors, giving you full control over your AI infrastructure.
Cons:
- Limited access to models: Most closed-source models like OpenAI's ChatGPT are not available for on-premise deployment. There are however an increasing number of open-sourced models like Mistral that can be used for commercial purposes.
- Significant upfront investment: Building and maintaining an on-premise solution can require substantial investment in hardware, software, and skilled personnel. This doesn't always have to be the case, as explained in another article on this blog about deploying LLMs with a web interface and an API locally in under 5 minutes.
- Maintenance and complexity: The responsibility for ongoing maintenance, updates, and scaling the infrastructure falls entirely on your organisation, necessitating a dedicated IT team.
- Limited scalability: Scaling an on-premise solution can be more challenging and costly than cloud-based alternatives, potentially leading to resource constraints.
GDPR Considerations: On-premise hosting is ideal for GDPR compliance, as it allows you to control all aspects of data handling and storage. However, this also means you bear full responsibility for meeting GDPR’s stringent requirements, including data access controls, breach notifications, and upholding data subject rights.
Conclusion
Selecting the appropriate deployment option for AI models requires carefully balancing your organisation’s specific needs with considerations related to intellectual property, data privacy, regulatory compliance, and operational complexity. Opting for a vendor through an enterprise plan offers ease of use and scalability, but it may raise concerns about intellectual property leakage and data privacy risks. Hosting in your own cloud provides greater control and flexibility but necessitates significant technical expertise. On-premise hosting delivers the highest level of security and autonomy but demands substantial investment and ongoing management.
Most organisations choose to build their AI applications in their own cloud and region to maintain full control over data flow, including intellectual property, and to comply with data privacy legislation. Additionally, for specific use cases with lower risk, they may opt to purchase an enterprise plan for certain SaaS solutions like GitHub Copilot for coding or Microsoft Copilot for Office. This typically involves a thorough vetting of terms and conditions by their legal department and data privacy officer (DPO), along with strict guidelines on how these tools can be used. Organisations that prioritise control over their data, security, and adherence to stringent regulations, such as those in government and defence, legal firms, banks, insurances providers, or healthcare, are more likely to prefer hosting AI models on-premise.